Machine learning experts struggle to deal with "overfitting" in neural networks. Evolution solved it with dreams, says new theory.
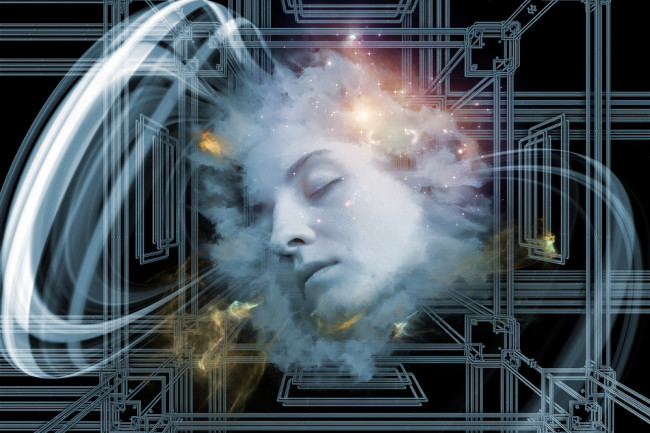
The world of sport is filled with superstition. Michael Jordan famously wore University of North Carolina shorts under his Chicago Bulls kit; Serena Williams wears the same socks throughout a tournament; and when Goran Ivanisevic won a tennis match, he would repeat that day’s activities throughout the competition.
Exactly the same thing occurs with artificial neural networks. The networks learn relevant detail but also irrelevances. Indeed, overfitting is the bane of machine learning experts who have devised a wide range of techniques to get around it.
All of this begs the question of how the human brain deals with overfitting. Our day-to-day experience can be hugely repetitive, so how does the brain generalize from these singular experiences to other situations?
Today, we get an answer thanks to the work of Erik Hoel, a neuroscientist at Tufts University in Massachusetts. Hoel’s answer is that the human brain prevents overfitting by dreaming. He says dreaming evolved specifically to deal with this problem, which is common to all neural networks. If his theory is correct, it answers one of the great unsolved problems in neuroscience: why we dream at all.
First, some background. Psychologists, neuroscientists and others have pondered the origin and role of dreams for time immemorial. Freud suggested that they were a way of expressing frustrations associated with taboos — an idea that has long been discredited.
Others have suggested dreams are a kind of emotional thermostat that allow us to control and resolve emotional conflicts. However, critics point out that most dreams lack strong emotional content and that emotionally neutral dreams are common.
Still others say dreams are part of the process the brain uses to fix memories or to selectively forget unwanted or unneeded memories. These theories also come up against the criticism that most dreams lack realistic detail, have a strange hallucinatory quality and often contain never-before-seen sequences. “Most dreams do not involve specific memories at all, making the integration of new memories a questionable purpose for dreaming,” says Hoel.
Machine Learning Clue
His new idea is that the purpose of dreams is to help the brain to make generalizations based on specific experiences. And they do this in a similar way to machine learning experts preventing overfitting in artificial neural networks.
The most common way to tackle overfitting is to add some noise to the learning process, to make it harder for the neural network to focus on irrelevant detail. In practice, researchers add noise to images or feed the computer with corrupted data and even remove random nodes in the neural network, a process known as dropout.
In human terms, this would be equivalent to forcing Michael Jordan to wear different combinations of shorts or making Serena Williams change her socks or Goran Ivanisevic adopting random routines on game days. This would make it much less likely that they would focus on a specific irrelevant detail.
Dreams perform the same function for the brain, says Hoel: “The purpose is to provide 'out-of-distribution' simulations specifically to prevent overfitting and improve generalization.”
He calls this idea the overfitted brain hypothesis and points out that there is plenty of evidence in its favor. For example, one of the best ways to trigger dreams is to embark on extensive sessions playing simple repetitive games such as Tetris. This creates the conditions in which the brain can become overfitted to task.
That’s why this kind of activity triggers dreams. These dreams are not replays of remembered Tetris games but tend to be sparse on detail with hallucinatory qualities. It is this “noise” that helps the brain generalize from the game. That’s also why people can improve their performance after a good night’s sleep.
Hoel uses his new theory to make a number of testable predictions. “It may be that direct measurement of overfitting is possible in humans,” he says. For example, one approach might be to train people in overly repetitive tasks and to see whether they can generalize this behavior, with and without sleep.
Dream Substitutes
The theory could also be used to better understand the types of mistakes that sleep-deprived individuals are likely to make and then to mitigate against these. “If it is true that sleep-deprived brains are overfitted, they will be prone to make errors in stereotypical ways,” he says.
Hoel says the theory suggests a way to treat sleep deprivation. “There is also the possibility of dream substitutions, wherein artificial dream-like stimuli might help improve generalization and therefore performance in sleep-deprived individuals,” he says.
The nature of dream substitutes is itself fascinating. Hoel says that fiction in general — books, plays, films etc — might perform a similar role to dreams. “They are, after all, explicitly false information,” he points out.
Just why humans create and enjoy fiction has always been something of a puzzle. But Hoel has an answer: “The overfitted brain hypothesis suggests fictions, and perhaps the arts in general, may actually have an underlying cognitive utility in the form of improving generalization and preventing overfitting, since they act as artificial dreams.”
That’s interesting work! Until now, most cognitive theories treat dreams as an epiphenomenon, an amusing by-product of sleep with no significant function of its own.
Hoel’s ideas turn all this upside down by providing a biological function for dreams and hence a rationale for their evolution for the first time.
Time for nap!
Ref: arxiv.org/abs/2007.09560 : The Overfitted Brain: Dreams Evolved to Assist Generalization