New models used writing samples to predict the onset of the disease with 70 percent accuracy
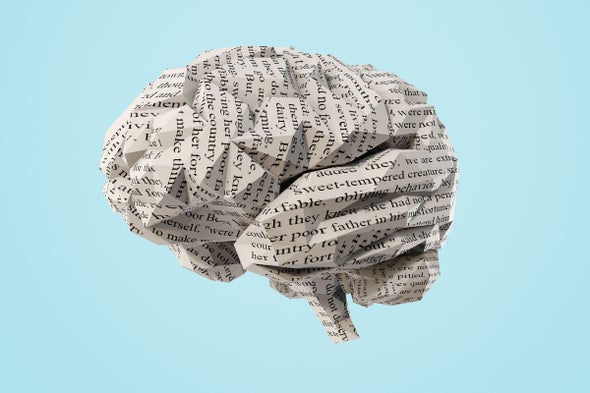
Artificial intelligence could soon help screen for Alzheimer’s disease by analyzing writing. A team from IBM and Pfizer says it has trained AI models to spot early signs of the notoriously stealthy illness by looking at linguistic patterns in word usage.
The new AI models provide “an augmentation to expert practitioners in how you would see some subtle changes earlier in time, before the clinical diagnosis has been achieved,” says Ajay Royyuru, vice president of health care and life sciences research at IBM. “It might actually alert you to some changes that [indicate] you ought to then go do a more complete exam.”
To train these models, the researchers used digital transcriptions of handwritten responses from Framingham Heart Study participants who were asked to describe a picture of a woman who is apparently preoccupied with washing dishes while two kids raid a cookie jar behind her back. These descriptions did not preserve the handwriting from the original responses, says Rhoda Au, director of neuropsychology at the Framingham study and a professor at Boston University. (Her team was responsible for transcribing data for the new paper but did not participate beyond that.) Yet even without the physical handwriting, IBM says its main AI model was able to detect linguistic features that are sometimes related to early signs of cognitive impairment. They include certain misspellings, repeated words and the use of simplified phrases rather than grammatically complex sentences. This evidence is in line with clinicians’ understanding of how Alzheimer’s disease can impact language, Royyuru says.
The main model achieved 70 percent accuracy in predicting which of the Framingham participants eventually developed dementia associated with Alzheimer’s disease before the age of 85. This result was based on historical data rather than actually predicting future events, however—and there are other caveats to the new paper as well.
The AI focused on the oldest group of Framingham study participants, who mostly represent a non-Hispanic white population. This limits how much the results can be generalized to more diverse communities in the U.S. and the rest of the world, Au notes. It also remains unclear how the AI would perform in larger populations: the EClinicalMedicine study’s data set involved just 40 people who eventually developed dementia and 40 “controls” who did not, notes Jekaterina Novikova, director of machine learning at Winterlight Labs in Toronto. Novikova, who was not involved in the new study, also questions whether the performance of IBM’s AI would change when predicting the onset of Alzheimer’s at different points in time prior to diagnosis.
Still, she and Au praise the paper as a solid contribution to the field that might draw more attention and resources to AI detection of Alzheimer’s. “What I like personally about the [study] is that it’s one of the very few works that analyzes the big-scale, real-life data that was collected over a very long period of time,” Novikova says.
The new models might have been more accurate if it could have incorporated handwriting, Au suggests. This ability could have provided additional clues, such as evidence of tiny tremors, switching between print and cursive, and very tiny letters. “There are a lot of ... features that [the researchers] did not account for, which, combined with linguistic features, would have probably created an even more predictive model,” Au says. The IBM models also did not include data from spoken language. Using AI speech analysis to diagnose Alzheimer’s is a growing area of research, and other systems have focused on detecting changes in audio samples. These contain clues such as speech pauses, which are not found in writing.
Whether written or spoken, language samples offer a relatively noninvasive source of information for monitoring peoples’ cognitive health, compared with brain scans and other laboratory tests. Collecting such language data could be done cheaply and remotely—though doing so would still require strict informed consent and privacy safeguards for the individuals creating the samples, Royyuru says. This is especially true because some people may not want to even know how likely they are to develop Alzheimer’s disease—a condition that is currently irreversible.
Training models on spoken rather than written samples might prove more practical for achieving the broadest reach in the long run, given that writing requires literacy while speech does not. Novikova and her colleagues at Winterlight Labs have been focusing heavily on teaching AI to analyze the acoustic and linguistic characteristics in spoken words. And Au has been recording both speech and handwriting, using digital pens to capture the latter, for her research. IBM seems to be thinking along the same lines for its own future work.
“We are in the process of leveraging this technology to better understand diseases such as schizophrenia, [amyotrophic lateral sclerosis] and Parkinson's disease and are doing so in prospective studies [that] analyze spoken speech samples, given with consent from similar cognitive verbal tests,” says Guillermo Cecchi, a co-author of the new study and a principal researcher for computational psychiatry and neuroimaging at IBM.